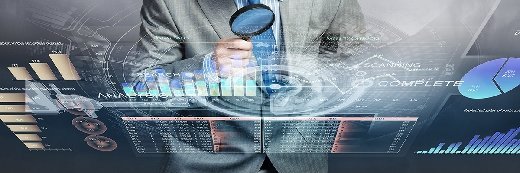
Sergey Nivens - Fotolia
Bringing business leaders, data science and advanced analytics together
How do you bridge the gap between academia and industry when it comes to data science and advanced analytics? Sai Devulapalli, Data Analytics Product and Business Leader at Dell, has some ideas.
Panelists at the 2017 Data Science conference in North Texas spent quite a bit of time exploring the gap between academia and industry when it comes to data science. But there is an even more important divide present within most for-profit organizations. That's the chasm that separates technologists and business leaders. As with any new approach to technology, internal analytics champions must be able to make a strong case if there is to be any hope for effective implementation with real results. How can this conversation be more effective?
Sai Devulapalli, Data Analytics Product and Business Leader at Dell, offered guidance in his presentation about Helping Business Leaders Get over Their Learning Curve in Advanced Analytics. In this informative talk, he uncovered the issues business stakeholders really care about and what data science and data analytics adoption looks like as an organization matures in its relationship to advanced analytics.
Businesses are ready to embrace analytics
Devulapalli got his start in data science in the 1990s. While some things have changed since, others have stayed the same. "The interesting fact is that a lot of the algorithms we used back then are still very relevant today. What has changed is the sophistication of the tools, the availability of more data, and the general awareness in the industry of, 'We've got to do something about this or the competition will.'"
In other words, businesses aren't asking whether they should be using analytics. They are more interested in figuring out how to get the biggest return with the lowest risk. When they turn to IT for answers, they need to understand what they hear. To communicate effectively, create alignment, obtain funding for projects, and ensure a successful implementation, technology leaders must realize how senior managers and executives think about the topic.
According to Sai, the most common questions business leaders have about analytics projects are:
- What is the ROI?
- What are the hidden costs?
- When will the project be delivered?
- How can I trust technology to make decisions?
- What business or organizational process changes must occur?
The answers depend on the use case, but there are some general rules that apply.
How much will it cost and what will the return be?
Sai admitted up front, "This is one of the toughest questions. None of the large consulting companies have a straightforward answer. It helps to look at it kind of like the stock market. There is a tradeoff between risk and reward. With stocks you have a higher risk but higher chance of reward. With bonds, there is lower risk but lower return."
The ROI is easiest to calculate with cost and risk mitigation projects. The cost of production is known and there are already risks present. "These projects can be very focused and narrow. You know what problem you are solving. But where a lot of companies drop the ball is progressing from the predictive model to the cost savings model. Companies that are mature in their thinking build a cost savings model based on the predictive model. Based on that, they determine the fixed costs and the lifecycle costs of the project and determine the ROI." Sai revealed that projects he's been involved with that fit this profile typically have an ROI of 50-300 times. "That's a really wide range based on the use case, but it's still quantifiable."
In contrast, revenue generation or innovation projects are often top down use cases. "Businesses say, 'We have this new market that we want to expand into, so let's think of some use cases and build out some predictive models and revenue models.' Those are more open ended and that's why it's so hard to actually come up with meaningful revenue models. That's big question mark." This is one reason Sai recommends starting with cost and risk mitigation projects.
Hidden costs and time to delivery
While initial costs may be straightforward to calculate, nothing stays static in an analytics project. "Lifecycle costs for managing an analytical model are often overlooked. That's because they are built on assumptions that are driven by environmental factors, and those factors always change. We have to constantly look at the model and update it." Underestimation is common.
As for the delivery timeline, that should be a straightforward question to answer simply because the tolerance for upper management is pretty well-established. "Six months to ROI is the typical comfort level." Projects should be built around milestones that provide a return within this timeframe or they are unlikely to get funded.
Building trust in analytics takes time
How can executive management start trusting advanced analytics? Convincing a business leader to hand over some of their decision-making to a machine is a tough sell. They may have relied on their intuition for decades. In fact, Devulapalli said it is possible to tell how far a company has come with its analytics processes by how much faith they put in the system.
"IBM originally proposed a maturity model with predictive and prescriptive models and there has been a lot of confusion and debate over the difference. The reason it matters is you can evaluate the trust management places in analytics projects based on these terms." With a predictive approach, business leaders will constantly check the model against their own intuition and reasoning. They apply analytics to a small section of the target business entity such as a particular product or a subset of the customer base. Typical predictive analytics might include looking at how to expand into a new area, reduce churn, or make a change in the product portfolio.
This limited approach is indicative of a lack of trust. As a result, the model requires a lot of oversight. But, in Sai's words, "After the model grows up, it is prescriptive. It doesn't require as much babysitting. It is learning how the environment is changing, and is targeting a larger segment such as 80% of your customer base." It's alright to start small and mature over time. That's simply the natural progression as business leaders get more comfortable with giving analytics a seat at the grownup table.
Data science and organizational structures
In an organization that's not yet doing advanced analytics in a big way, it's normal for the initiative to start off with IT and different business units and then make the way up the ladder to corporate. The big problem tends to be getting the data organized. "The stakeholders are trying to get a couple of use cases addressed. Then they find out their data is spread out across multiple data stores, warehouses, and vendors. When you start sharing data across these different sources, you run into an issue of integrity, security, ownership, and management. It's necessary to do some sort of federation." The changes that must occur are often both procedural and technological.
At a higher level of maturity, a data analytics initiative is often driven from the management side. The primary stakeholder may be a VP or Director of Analytics with different business units and entities arranged in a hierarchical structure. Data management across the organization may already be in a state to be readily available for analytics projects. Sai pointed to the advantages of this system. "The more sources of information you have, the better the predictive ability." Economies of scale come into play, and data ownership boundaries become less significant. The analytics process can simply acquire and use a copy of the data rather than owning it all.
Core message for analytics advocates within the organization
The takeaways for stakeholders driving analytics projects were simple. They should always speak in terms of business value rather than techno-jargon, present a couple of sensible use cases rather than proposing an open ended data fishing expedition, and put a clear timeline on the project. Whenever possible, they should provide reasonable estimates on cost and ROI while acknowledging that hidden costs and opportunities may exist. They should also acknowledge the expertise and savvy that business leaders bring to the table and expect change to happen in stages rather than all at once. Analyzing the playing field and the players before proposing an advanced analytics project will make it much more likely to succeed.