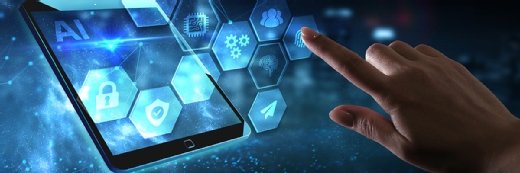
putilov_denis - stock.adobe.com
Get started with Java and AI: A guide to LLM integration
Want to unlock the power of AI in your Java applications? This walkthrough guides you on how to use OpenAI and Java with large language models to unlock powerful new capabilities.
Integrating large language models into Java applications unlocks powerful capabilities, ranging from intelligent automation to sophisticated conversational interfaces. This guide explores how to use LangChain4j and OpenAI to create a simple yet effective CLI-based Java application. Think of this as the "Hello World" of Java and AI/LLM integration.
You will need several prerequisites for this tutorial:
- Java 21 (this example doesn't depend on a specific JDK distribution, but you can also use the latest one).
- Internet connectivity to communicate with OpenAI's API.
- OpenAI API key for authentication.
Obtain an OpenAI API key
Getting your OpenAI API key is straightforward. Follow these steps:
- Sign up or log in. Go to OpenAI and create an account or log in if you already have one.
- Navigate to the API Keys section. Look for your account dashboard's "API Keys" section.
- Generate and securely store your API key. Click the button to generate a new API key. Make sure to store it in a safe place, as you won't be able to see it again.
Set up your environment
Store your API key securely as an environment variable. Here's how, depending on what OS you're using:
- On Windows, navigate to: System Properties > User Variables > Add OPENAI_API_KEY.
- On macOS or Linux, add export OPENAI_API_KEY='your-api-key' to your .bashrc or .zshrc file.
Simple CLI example: The 'Hello World' of Java and LLM
Let's start with a minimal example that demonstrates how to use Java and OpenAI's GPT model using a command-line interface. This example uses Java's built-in HTTP client to make API requests to OpenAI.
package ca.bazlur;
import java.net.http.*;
import java.net.URI;
import java.time.Duration;
public class Main {
private static final String API_URL = "https://api.openai.com/v1/chat/completions";
public static void main(String[] args) throws Exception {
String apiKey = System.getenv("OPENAI_API_KEY");
if (apiKey == null || apiKey.isBlank()) {
System.err.println("Error: OPENAI_API_KEY environment variable not set.");
System.exit(1);
}
if (args.length == 0 || args[0].isBlank()) {
System.err.println("Error: Please provide a prompt.");
System.exit(1);
}
String prompt = args[0];
String requestBody = """
{
"model": "gpt-4",
"messages": [{"role": "user", "content": "%s"}],
"temperature": 0.7,
"max_tokens": 150
}
""".formatted(prompt);
try (HttpClient client = HttpClient.newHttpClient()) {
HttpRequest request = HttpRequest.newBuilder()
.uri(URI.create(API_URL))
.header("Content-Type", "application/json")
.header("Authorization", "Bearer " + apiKey)
.POST(HttpRequest.BodyPublishers.ofString(requestBody))
.timeout(Duration.ofSeconds(30))
.build();
HttpResponse<String> response = client.send(request, HttpResponse.BodyHandlers.ofString());
System.out.println("ChatGPT Response: " + response.body());
}
}
}
In the above code, we constructed the request body as a JSON string and specified the model (gpt-4), the user's message, temperature and the maximum number of tokens. The model parameter specifies which OpenAI model to use; gpt-4 is a powerful and versatile option. The messages array contains the conversation history, and the role parameter indicates who sent the message. Temperature and maximum tokens are crucial to control the output.
The temperature parameter, which ranges from 0 to 1, influences the randomness of the generated text. A higher temperature makes the output more creative and unpredictable, whereas a lower temperature results in a more conservative and predictable output. For instance, asking "Tell me a joke" with a temperature of 0.2 might produce a classic well-known joke, while a temperature of 1.0 could generate a more absurd and original response though it might be less coherent.
The max_tokens parameter limits the length of the generated text, which is crucial for managing costs and preventing excessively lengthy responses. If you set max_tokens to 50, the model will produce a response that does not exceed 50 tokens. Setting it too low might truncate the response prematurely, while setting it too high could result in verbose or rambling answers.
We then created an HttpClient to send the API request, and built an HttpRequest with the API endpoint, headers (Content-Type and Authorization) and request body. We sent the request using client.send(), retrieved the response and finally printed the response body to the console.
Here's how to run the code from the following example:
- Save the code as Main.java.
- Run the code: java Main.java "What is the capital of Toronto?"
And we get the following output:
{
"id": "chatcmpl-BDTz5FwsX1rAfcjQZYP64JBWuUopl",
"object": "chat.completion",
"created": 1742553223,
"model": "gpt-4-0613",
"choices": [
{
"index": 0,
"message": {
"role": "assistant",
"content": "Toronto is a city, not a country, so it doesn't have a capital. It is the capital city of the province of Ontario in Canada.",
"refusal": null,
"annotations": []
},
"logprobs": null,
"finish_reason": "stop"
}
],
"usage": {
"prompt_tokens": 14,
"completion_tokens": 31,
"total_tokens": 45,
"prompt_tokens_details": {
"cached_tokens": 0,
"audio_tokens": 0
},
"completion_tokens_details": {
"reasoning_tokens": 0,
"audio_tokens": 0,
"accepted_prediction_tokens": 0,
"rejected_prediction_tokens": 0
}
},
"service_tier": "default",
"system_fingerprint": null
}
That JSON payload contains information such as usages, the model used, the creation timestamp, and the actual message content from the assistant. We can then parse it using a JSON parser and extract what we need.
Integrating with LangChain4j (OpenAI SDK)
While the previous example demonstrates a direct approach to interacting with the OpenAI API, it involves manually constructing HTTP requests and parsing JSON responses. This can become cumbersome and error-prone, especially when building complex applications.
The Java-native library LangChain4j simplifies this process. It provides a high-level, intuitive API to interact with LLMs such as OpenAI's GPT models, abstracting away the underlying complexities of API calls so developers can focus on the application's logic.
LangChain4J OpenAI example
Let's set up a simple Maven project or Gradle project with several dependencies. Here's how that would look in a Maven pom.xml:
<dependency>
<groupId>dev.langchain4j</groupId>
<artifactId>langchain4j</artifactId>
<version>1.0.0-beta2</version>
</dependency>
<dependency>
<groupId>dev.langchain4j</groupId>
<artifactId>langchain4j-open-ai</artifactId>
<version>1.0.0-beta2</version>
</dependency>
Or, for build.gradle:
implementation 'dev.langchain4j:langchain4j:1.0.0-beta2'
implementation 'dev.langchain4j:langchain4j-open-ai:1.0.0-beta2'
Example with LangChain4j
Now, let's create a Java class that uses LangChain4j to interact with OpenAI:
import dev.langchain4j.model.chat.ChatLanguageModel;
import dev.langchain4j.model.openai.OpenAiChatModel;
public class LLMExample {
public static void main(String[] args) {
ChatLanguageModel model = OpenAiChatModel.builder()
.apiKey(System.getenv("OPENAI_API_KEY"))
.modelName("gpt-4")
.temperature(0.7)
.maxTokens(150)
.build();
String response = model.chat("What is the capital of Toronto?");
System.out.println(response);
}
}
In that code, we created an instance of OpenAiChatModel using the builder pattern. We set the API key, model name, temperature and maximum number of tokens. As in the prior example, the temperature parameter influences the randomness of the generated text to be conservative and predictable or more creative but less coherent, while max_tokens prevents excessively long responses as a cost-control measure. Experiment with these parameters to significantly alter the LLM's output and tailor it to your specific needs.
Calling the chat() method on the model passes in the user's prompt, the method returns the generated text, and finally we print the response to the console.
To run this example, we perform two steps:
- Save the code as LLMExample.java in the src/main/java directory of your Maven/Gradle project.
- Compile and run the code using Maven: mvn compile exec:java -Dexec.mainClass="LLMExample"
Alternative, to run this with Gradle, use the command: ./gradlew run
You should see a response from ChatGPT printed on your console.
LangChain4j and other AI models
Besides OpenAI, LangChain4j supports integration with other LLMs so developers can experiment with different LLMs and choose the one that best suits their needs. For example, if we want to use Google's Gemini API, we adjust the code to change the base URL and model name, as so:
import dev.langchain4j.model.chat.ChatLanguageModel;
import dev.langchain4j.model.openai.OpenAiChatModel;
public class LLMGeminiExample {
public static void main(String[] args) {
ChatLanguageModel model = OpenAiChatModel.builder()
.apiKey(System.getenv("GOOGLE_API_KEY"))
.baseUrl("https://generativelanguage.googleapis.com/v1beta/openai/")
.modelName("gemini-2.0-flash")
.temperature(0.7)
.maxTokens(150)
.build();
String response = model.chat("What is the capital of Toronto?");
System.out.println(response);
}
}
Of course, you must obtain the Gemini API key, which you can get from Google AI Studio. Make sure you store it in an environment variable.
Also, notice that we used the same OpenAI model. In fact, this is the standard SDK for most of the models so that we can use it uniformly.
The source code for these Java and OpenAI LLM examples is available on GitHub.
What to do next with Java, OpenAI and LLMs
Now that you've created your first Java and LLM application, you can expand this basic setup to explore deeper integrations and build more sophisticated AI-powered Java applications. Here are some ideas for further exploration:
- Build a chatbot. Create a simple chatbot to answer user questions about a specific topic.
- Implement text summarization. Use an LLM to summarize long articles or documents.
- Generate creative content. Experiment with using LLMs to generate poems, stories or code.
- Explore different AI models. Try integrating with other LLMs, such as Anthropic API.
With some practice and confidence, you'll be ready to explore advanced topics around Java and AI, including optimizations and practical LLM implementations in Java.
A N M Bazlur Rahman is a Java Champion and staff software developer at DNAstack. He is also founder and moderator of the Java User Group in Bangladesh.