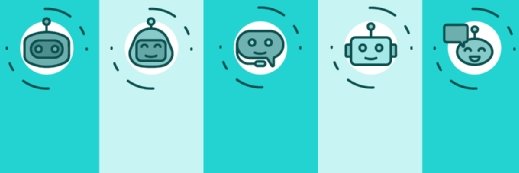
How to build an AI agent tutorial with example
How do AI agents work? Are they like cron jobs? This walkthrough explains what AI agents can do, the basic principles that apply, and how to build your own AI agent using ChatGPT.
An AI agent is software that uses large language models and similar technologies to execute behavior automatically and intelligently for a specific purpose. It can be used on demand, such as Amazon's Alexa or Apple's Siri, although some consider these strictly AI assistants that require and react to user input. An AI agent can also be used in a manner that's similar to a cron job, to run at a specific regular recurrence or even nonstop.
What distinguishes an AI agent from other types of software is its heavy dependence on AI technologies, and its ability to run and change behavior with a minimal amount of human intervention.
Interest in AI agents and their usefulness across many applications is growing fast. This AI agent tutorial presents the basic principles that govern AI agents, with a demonstration application example of how to build an AI agent.
The basic principles
One way to think of an AI agent is that it is similar to a human talent or professional agent who works to promote the client's interests.
Talent agents have a great deal of autonomy in how they do their job. They find suitable work on behalf of the client and negotiate compensation and payment. The agent interacts with the client only at key decision points, such as whether to take an acting role or accept a contract at a certain pay rate. All other matters are left up to the agent.
The same can be said about an AI agent. Technical considerations aside, the key principles that drive an AI agent are as follows:
Logical autonomy. AI agents operate independently and make decisions based on their environment. Also, an AI agent needs to be able to adjust its logic over time as its learning and awareness increase.
Ability to process unstructured data. AI agents can process unstructured data and interpret that data in a structured, reportable manner.
Natural language processing. AI agents can interact with users and other systems through natural language processing, rather than making statements governed by the syntax of a particular programming language.
Collaboration and scalability. An AI agent can interact with other agents using natural language prompts. Also, an AI agent can scale its processing capability up and down to meet momentary needs.
Minimal manual intervention to maintain. AI agents can adapt to changes in their environment with minimal manual intervention, which reduces maintenance efforts.
Let's look at a demonstration application to get a sense of how to program an AI agent. The code and results described in this example are available on GitHub.
Programming with natural language prompts
This demonstration application is an AI agent written in TypeScript. Its purpose is to continuously report five things to do in a city that has the best weather at the time of inquiry on a global or regional basis.
As shown in the logical diagram below (Figure 1), the agent has three high-level activities. First, the agent queries the ChatGPT API to list the most visited 20 cities in a region (callout 1). The application's code supports a global region (worldwide) as well as the regions enumerated as NorthAmerica, Europe, Asia, MiddleEast, Africa, SouthAmerica, and Oceania.
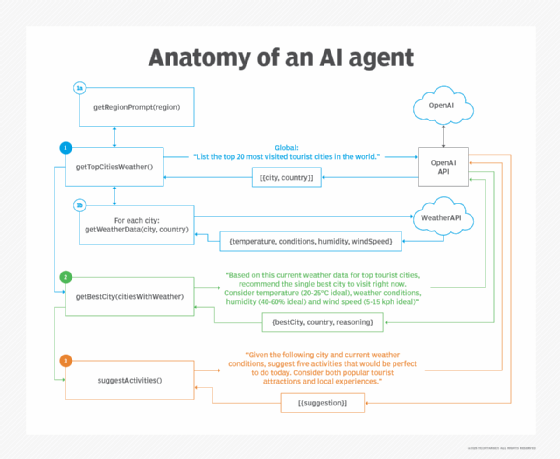
Once the 20 cities are retrieved, a call is made to an online weather API to determine the current weather in each city in the list of 20 (callout 1b). Then, a call is made to ChatGPT to determine the best city in the list of 20 according to the current weather in the city (callout 2). Finally, a call is made to the ChatGPT API to list five things to do in the city identified to have the best weather (callout 3).
The following response shows the result when the agent executes its logic according to the region NorthAmerica.
Starting hourly travel monitoring for NorthAmerica...
Checking travel recommendations for NorthAmerica at 2/16/2025, 3:01:02 PM
=== New Travel Recommendation for NorthAmerica ===
Best City: Los Angeles, USA
Reasoning: Los Angeles offers the most ideal weather conditions based on the specified criteria. The temperature in Los Angeles is 21.1°C, which falls within the ideal range of 20-25°C. The weather is sunny, providing a pleasant atmosphere for outdoor activities and sightseeing. Although the humidity is slightly below the ideal range at 28%, it is still comfortable for most people. The wind speed is 9 kph, which is within the ideal range of 5-15 kph, ensuring that it's not too calm or too windy. Considering all these factors, Los Angeles stands out as the best city to visit right now.
Top 5 Things to Do Today:
1. Take a walk along the Santa Monica Pier and enjoy the ocean views.
2. Explore the Griffith Observatory for stunning city views and a closer look at the stars.
3. Visit the Getty Center to enjoy world-class art collections and beautiful garden areas.
4. Spend the day at Venice Beach, experiencing the unique street performers and the vibrant skateboarding culture.
5. Hike to the Hollywood Sign for an iconic Los Angeles experience and a great photo opportunity.
================================
As shown in the figure above, the demonstration AI agent depends on ChatGPT and a weather API to obtain the information required to fulfill its purpose. The AI agent interacts with ChatGPT through natural language prompts.
The next code block below shows the function getRegionPrompt(region: Region), which returns a string that is a natural language prompt asking for the most visited cities in a particular region.
private getRegionPrompt(region: Region): string {
const regionPrompts = {
Global: "List the top 20 most visited tourist cities in the world.",
NorthAmerica: "List the top 20 most visited tourist cities in North America (including USA, Canada, and Mexico).",
Europe: "List the top 20 most visited tourist cities in Europe.",
Asia: "List the top 20 most visited tourist cities in Asia (excluding Middle East).",
MiddleEast: "List the top 20 most visited tourist cities in the Middle East.",
Africa: "List the top 20 most visited tourist cities in Africa.",
SouthAmerica: "List the top 20 most visited tourist cities in South America.",
Oceania: "List the top 20 most visited tourist cities in Oceania (including Australia and New Zealand)."
};
return regionPrompts[region];
}
Once the program has a list of cities, either globally or by region, the code sends the name and country of each city in the list to the Weather API using the function getBestCity(citiesWithWeather: any[]) to get the city's current weather. The weather for each city is added to a list which is then sent to ChatGPT as a prompt to determine the best city to visit according to the current weather. The prompt is composed of all the cities to consider, as well as the structure of the information to be returned to the caller.
The prompt that is created is shown in the following code block.
const prompt = `Based on this current weather data for top tourist cities, recommend the single best city to visit right now. Consider temperature (20-25°C ideal), weather conditions, humidity (40-60% ideal), and wind speed (5-15 kph ideal).
Cities and their current conditions:
${citiesWithWeather.map(city => `
${city.name}, ${city.country}:
- Temperature: ${city.weather.temperature}°C
- Weather: ${city.weather.conditions}
- Humidity: ${city.weather.humidity}%
- Wind Speed: ${city.weather.windSpeed} kph
`).join('\n')}
Return your response in this JSON format:
{
"bestCity": "city name",
"country": "country name",
"reasoning": "detailed explanation of why this city is the best choice right now"
}`
Once the best city is determined according to the current weather, another trip is made back to ChatGPT. Only this time, the code asks ChatGPT to suggest five activities to do in the identified city.
The following code block shows the code for the function suggestActivities(cityName: string, countryName: string,weatherConditions: string), which creates the prompt to submit to ChatGPT. The prompt not only makes the request for the five activities to do in the city but also tells ChatGPT to return the information as a JavaScript array of strings.
public async suggestActivities(cityName: string, countryName: string,
weatherConditions: string): Promise < string[] > {
try {
const prompt = `Given the following city and current weather conditions, suggest 5 activities that would be perfect to do today. Consider both popular tourist attractions and local experiences.
City: ${cityName}, ${countryName}
Current weather: ${weatherConditions}
Return your response as a JSON array of strings, with each string being a suggested activity.`;
const response = await this.openai.chat.completions.create({
model: "gpt-4-turbo-preview",
messages: [{
role: "user",
content: prompt
}],
response_format: {
type: "json_object"
},
temperature: 0.7
});
const activities = JSON.parse( < string > response.choices[0].message.content).activities;
return activities;
} catch (error) {
console.error('Failed to get activity suggestions:', error);
throw new Error('Could not generate activity suggestions');
}
}
All the code listings up to this point represent the building blocks that make up the AI agent. However, to make the agent run without user intervention, we must implement one final piece of logic.
The following code block shows a snippet of code from checkAndRecommend(), which is the function that puts it all together to create the overall logic of the agent.
const citiesWithWeather = await this.getTopCitiesWithWeather(region);
const recommendation = await this.getBestCity(citiesWithWeather);
// Get activity suggestions for the recommended city
const activities = await this.suggestActivities(
recommendation.bestCity,
recommendation.country,
citiesWithWeather.find(c => c.name === recommendation.bestCity)?.weather.conditions || 'good weather'
);
And here is the final piece of the JavaScript code that keeps the agent running; the agent will check for activities once a day.
async function runTravelMonitor() {
const monitor = new HourlyTravelMonitor();
// Start monitoring
await monitor.start('NorthAmerica');
// Optional: Stop after 24 hours
setTimeout(() => {
monitor.stop();
console.log('Monitoring stopped after 24 hours');
}, 24 * 60 * 60 * 1000);
}
// Run the agent
runTravelMonitor().catch(console.error);
Calling the function runTravelMonitor() ensures that the agent reports five things to do in the city in a given region, given its current weather. That final block of code instructs the agent to constrain its analysis to cities in North America.
Putting it all together
This demonstration project to generate and deploy an AI agent is simple, but there are significant opportunities for improvement.
First, the region by which cities with the best weather are determined is hardcoded. Instead, one could allow the user to provide an initial prompt to the agent. For example, the agent could ask, "Which regions on the planet do you want me to inspect?" Then the user could respond, "Show me five activities to do in the city in North America and Europe that have the best current weather." This feature would make the agent more flexible. Furthermore, we could let the user declare the number of activities to return, which would make the agent even more flexible.
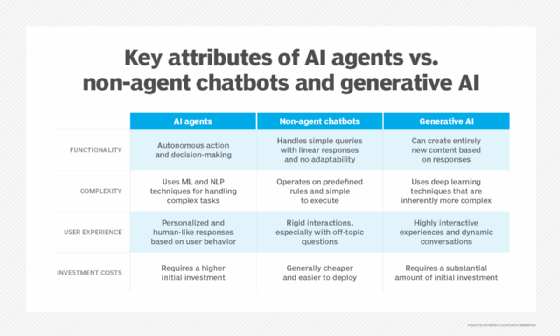
Second, the agent is unable to modify its behavior as it encounters unexpected or questionable results. It simply errors out. This is OK in the short run, but to ensure the application's long-term viability, it must implement and maintain the principle of supporting minimal manual intervention.
For a start, we would need to rewrite the code just to change the frequency by which activities for the best city are determined, from once a day to a user-determined period. To implement this fix according to the principles described above, the agent asks the user for the frequency at which the activities should be reported, and then the user replies accordingly. Once the parameters are established, the agent can run independently, requiring human intervention only when the user wants to change the operating parameters.
At a more advanced level, a comprehensive AI agent could, for example, determine its shortcomings, not let the user determine the regions to inspect and the frequency at which inspection occurs, and adjust its code accordingly. This greater level of complexity requires advanced programming skills. Nevertheless, this demonstration project serves as a practical illustration of how to build an AI agent. Your growing skills and imagination will make it better.
Bob Reselman is a software developer, system architect and writer. His expertise ranges from software development technologies to techniques and culture.