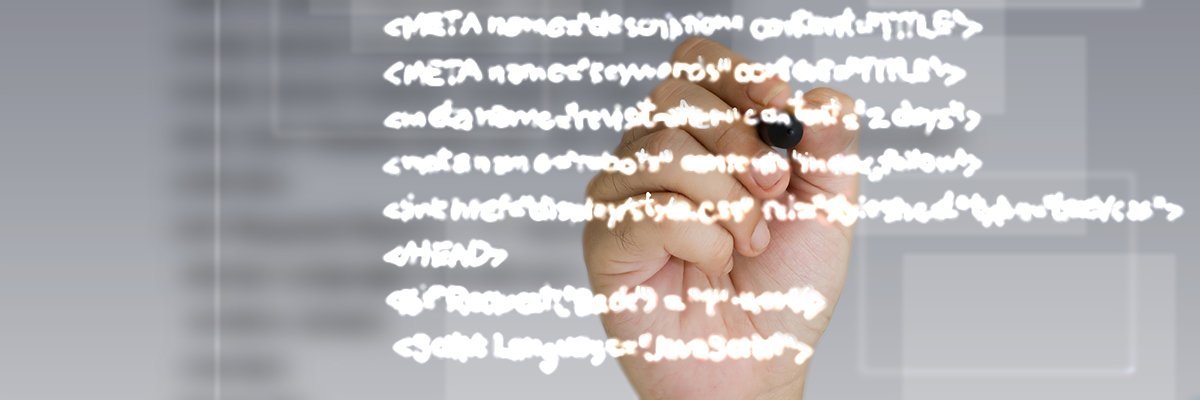
patpitchaya - Fotolia
Coffee Talk: Java, News, Stories and Opinions
Community driven content discussing all aspects of software development from DevOps to design patterns.
Recent Posts
-
How to create an account alias in AWS
- TechTarget 07 Jun 2025 -
Obsolete vs. deprecated in software development
- TechTarget 21 May 2025 -
Component vs. Bean annotations in Spring
- TechTarget 20 May 2025
Need to create, change or rename an AWS account alias? It's not too hard to do, and your users will thank you. Here are the steps to do it.
Ever wonder what is the difference between an obsolete component and a deprecated component? Here's the answer in the most concise terms you'll find.
Ever wonder what sets the Spring @Component and @Bean annotations apart? The difference is subtle, but for Spring Boot developers, it's an important distinction to know.
-
How to convert Figma designs to code with Cursor AI
- Scrumtuous Inc. 13 May 2025 -
How to use Swagger with Spring Boot
- TechTarget 07 May 2025 -
Deprecated vs depreciated: What's the difference?
- TechTarget 04 May 2025 -
Format output with Java printf
- TechTarget 30 Apr 2025 -
How to use git stash pop
- TechTarget 28 Apr 2025 -
Java, JDBC and the HSQLDB database tutorial
- TechTarget 20 Apr 2025 -
User input and Java's JOptionPane example
- TechTarget 19 Apr 2025
One of the biggest challenges design teams and web developers face is turning Figma designs into responsive, professional-looking web pages that remain faithful to the original vision. Fortunately, ...
The easiest way to build, test and document RESTful APIs built with Spring Boot? Simply add Swagger to your Spring Boot project and let OpenAPI do it for you.
Did you just hear someone say a method was depreciated? They probably mean deprecated. Here's the difference between the two.
Learn by example how format output with the Java printf method. If you want to format dates, times, integers, doubles and Strings in the text you output to the console, logs or streams, you'll need ...
To move shelved changes from the Git stash to your working directory, the git stash pop command is preferred over apply, as pop will delete the stash in question, keeping the stash history clean.
Here's a quick JDBC with HSQLDB tutorial to get you up and running with Java database connectivity and the popular HyperSQL database.
For new Java programmers, the JOptionPane class is a great visual and user-friendly introduction to user input and Swing-based programming. Here's how to get started.
-
What is Scrum? The Scrum process explained in 5 minutes
- Scrumtuous Inc. 07 Apr 2025 -
Is GitHub Copilot free?
- TechTarget 03 Apr 2025 -
How to delete a local Git branch
- TechTarget 30 Jan 2025 -
How to download and install Java 21 on Windows
- TechTarget 06 Jan 2025 -
How to install Java 21 on Ubuntu
- TechTarget 01 Jan 2025 -
The 5-second rule to create effective technical illustrations
- CogArtTech 12 Dec 2024 -
How to pitch to VCs: 5 questions a tech startup must answer
- CogArtTech 02 Dec 2024 -
What is Maven in Java? Crash course tutorial for beginners
- TechTarget 05 Nov 2024 -
Get started with Spring Boot 3 tutorial
- TechTarget 01 Nov 2024 -
Spring Boot file upload example
- TechTarget 27 Oct 2024 -
Pursuing the exit event: Why software startups aren't family businesses
- CogArtTech 24 Oct 2024 -
SQLite3 Basics: Create tables and insert records
- TechTarget 21 Oct 2024 -
How to install SQLite on Windows
- TechTarget 20 Oct 2024 -
Do AI capabilities enhance or impair human cognition?
- CogArtTech 15 Oct 2024 -
What is JDBC's full form?
- TechTarget 10 Oct 2024 -
Java's 4 JDBC driver types explained
- TechTarget 08 Oct 2024 -
Database transaction isolation levels tutorial
- TechTarget 07 Oct 2024 -
How do you change the Spring Boot startup banner?
- TechTarget 04 Oct 2024 -
How the Spring @Profile annotation works
- TechTarget 03 Oct 2024 -
Imperative Git commit messages in the active tense or mood
- TechTarget 02 Oct 2024 -
Benefits of Spring Boot's application.yml file with examples
- TechTarget 01 Oct 2024 -
Profiles, Spring Boot and application properties files
- TechTarget 30 Sep 2024 -
Code a tic-tac-toe game in Java
- TechTarget 18 Jun 2024 -
Git pull vs fetch: What's the difference?
- TechTarget 17 Jun 2024 -
Worlds toughest core Java interview question
- Scrumtuous Inc. 08 Apr 2024 -
What is MVCC? How does multiversion concurrency control work?
- TechTarget 04 Apr 2024 -
Best crash course to learn Jenkins from scratch
- TechTarget 04 Mar 2024 -
How to git push an existing project to GitLab
- TechTarget 01 Mar 2024 -
Brackets vs braces vs parentheses: What's the difference?
- Scrumtuous Inc. 27 Feb 2024 -
Set a Git stash message. Pop with a Git stash name
- TechTarget 25 Feb 2024 -
How to list and show the git stash history
- TechTarget 23 Feb 2024 -
Git branch rename: Command to change a branch name in Git
- TechTarget 21 Feb 2024 -
git@github: permission denied (PublicKey) SSH error fix
- TechTarget 16 Feb 2024 -
String to long in Java
- TechTarget 14 Feb 2024 -
How to unstage a file in Git
- TechTarget 02 Feb 2024 -
Why the Java const keyword is unimplemented
- TechTarget 01 Feb 2024 -
How to setup SSH in GitHub by example
- TechTarget 30 Jan 2024 -
Quickly generate, add and setup GitLab SSH Keys
- TechTarget 30 Jan 2024 -
How to find and use a GitHub URL by example
- TechTarget 25 Jan 2024 -
System, global and local Git config files on Windows and Linux
- TechTarget 19 Jan 2024 -
Finding duplicate entries in a list in Python
- Lairds Computer Services 18 Jan 2024 -
Solve the prefix sum problem with SIMDs and Vector APIs
- TechTarget 17 Jan 2024 -
Set your username and email in Git config
- TechTarget 15 Jan 2024 -
Install the Eclipse IDE on Windows video tutorial
- TechTarget 09 Jan 2024 -
Find duplicates in a List in Java
- Lairds Computer Services 09 Jan 2024 -
The prefix sum array problem
- TechTarget 08 Jan 2024 -
Remove duplicates from a Java List
- Lairds Computer Services 27 Dec 2023 -
How to code your first Mojo program
- Scrumtuous Inc. 17 Dec 2023 -
How the Actor Model works by example
- CogArtTech 06 Dec 2023 -
How to amend a git commit
- TechTarget 28 Nov 2023 -
The git add command for beginners
- TechTarget 19 Nov 2023 -
How to create a Git repository
- TechTarget 18 Nov 2023 -
Java vs. Python: Application code comparison
- Scrumtuous Inc. 18 Sep 2023 -
Is Python's GIL the software world's biggest blunder?
- Scrumtuous Inc. 12 Sep 2023 -
How to install Git on Windows
- TechTarget 05 Sep 2023 -
Clean Git up!
- TechTarget 05 Sep 2023 -
How to git clone a specific commit
- TechTarget 04 Sep 2023 -
How to git clone a specific branch
- TechTarget 02 Sep 2023 -
git clean: How to remove untracked files in Git
- TechTarget 01 Sep 2023 -
How to discard local changes in Git
- TechTarget 01 Sep 2023 -
Git push new local branch to remote
- TechTarget 31 Aug 2023 -
GitKraken tutorial for beginners
- TechTarget 31 Aug 2023 -
10 reasons to use Kotlin
- EnigmaStation 30 Aug 2023 -
How to git push an existing project to GitHub
- TechTarget 29 Aug 2023 -
Sourcetree tutorial for beginners
- TechTarget 29 Aug 2023 -
How to install Jenkins on Windows
- TechTarget 24 Aug 2023 -
GitHub Desktop Tutorial for beginners
- TechTarget 24 Aug 2023 -
Git reset hard vs. soft: What's the difference?
- TechTarget 23 Aug 2023 -
How to delete local and remote Git branches
- TechTarget 22 Aug 2023 -
HTTP request methods explained
- TechTarget 21 Aug 2023 -
PUT vs POST: What's the difference?
- TechTarget 21 Aug 2023 -
How to login to Docker with a Docker Hub access token
- TechTarget 07 Aug 2023 -
Sprint vs Scrum: What's the difference?
- Scrumtuous Inc. 19 Jul 2023 -
Create your first Python AWS Lambda function in minutes
- TechTarget 17 Jul 2023 -
How to enable Java 21 preview features
- TechTarget 13 Jul 2023 -
Scrum's chicken and pigs parable
- Scrumtuous Inc. 10 Jul 2023 -
Please make 'please' a sudo alias on your Linux box
- Scrumtuous Inc. 06 Jul 2023 -
Create your first Java AWS Lambda function in minutes
- TechTarget 03 Jul 2023 -
The var keyword in Java
- TechTarget 01 Jul 2023 -
Leibniz in Java and the Commodore 64: An exercise in optimization
- Lairds Computer Services 30 Jun 2023 -
How to pass a Scrum certification exam with a 100% score
- Scrumtuous Inc. 20 Jun 2023 -
How Java instance main methods and unnamed classes work
- TechTarget 06 Jun 2023 -
Hibernate and JPA column mapping with annotations
- TechTarget 04 Jun 2023 -
How System.out in Java works
- TechTarget 01 Jun 2023 -
How to use native SQL in Hibernate and JPA
- TechTarget 01 Jun 2023 -
Java's print vs println method: What's the difference?
- TechTarget 31 May 2023 -
Is the daily Scrum recommended for colocated teams?
- Scrumtuous Inc. 25 May 2023 -
Hibernate and JPA 3 CRUD example
- TechTarget 15 May 2023 -
How long is a Sprint in Agile?
- Scrumtuous Inc. 10 May 2023 -
Five ways to fix the 'no persistence.xml file found' error in Eclipse
- TechTarget 09 May 2023
The Scrum framework is the software development industry's most popular Agile methodology. Here are the key steps that define how the Scrum process flows.
GitHub Copilot pricing includes a basic free tier that provides code completions and chat requests. But does that mean it's free? Yes and no.
Here's a quick example of how to delete local Git branches from your workstation without deleting remote branches in your GitHub or GitLab repos.
2024 is a great time to start working with Java, because 2023's Java 21 release has some amazing new features. Here's how to quickly download and install the Java 21 JDK.
Here's the easiest way to install Java on Ubuntu with apt. Follow these steps and you'll get the JDK installed in no time, and be ready to use your Java on Ubuntu setup right away.
Like any picture, a well-crafted technical illustration is worth a thousand words. Make the most of your diagrams and flowcharts and follow the 5-second rule for reader usability.
Tech startups pitching investors for funding must quickly and convincingly present their business case. Prepare your answers to these five questions and you'll nail that meeting.
Need to learn Apache Maven fast? This crash course Maven tutorial will quickly get you up to speed on the popular, open-source, Java build tool.
Need to learn Spring Boot 3? Want to quickly build a Spring Boot app with RESTful web services? This tutorial will help you create your first Spring Boot application in just minutes.
Do you need to find a Spring Boot file upload that uses Ajax and works with Spring MVC web apps? This example uses HTML5 file input fields and a pure JavaScript Ajax call to accomplish the task.
Launching a software startup is doable, but know what you're getting into with long-term goals. There are few mom-and-pop software shops for good reasons.
The SQLite3 command line interface makes it incredibly easy to create databases, configure tables, delete records and even get status updates on the state of your SQLite database. If you're using ...
SQLite is as easy to install on Windows as it is to use. Simply download the precompiled binaries and tools from sqlite.org, extract them to a sensible folder and add that folder to the PATH of the ...
AI has many benefits and uses, but we should be cautious where there is potential crossover in which AI capabilities undercut human learning abilities and skills.
Some argue the full form of JDBC is Java Database Connectivity, while others argue it is a trademarked term and doesn't actually stand for anything. So which side of the debate is right?
Here we explain the difference between the four JDBC driver types, and how to choose the right JDBC driver for your database-driven Java apps.
Repeatable? Isolated? Serializable? Uncommitted? None? Just how well do you know your database isolation levels, and how do you know if you're using the right one? Here's how you know.
Tired of seeing that Spring Boot banner pop up every time you redeploy your microservices? Here's how to turn it off. Or even better, customize it to your liking.
Everyone is used to configuring multiple properties files to support Spring profiles, but the Spring @Profile annotation allows you to control the Spring's dependency injection behavior at runtime too.
The pundits all say Git commit messages should be written in the imperative tense. I say that's outdated thinking.
Are you still stuck in the dark ages, configuring your Spring Boot apps with application.properties files? You really should start using an appliation.yml file. Here's just a few of the benefits ...
Spring profiles are a powerful and flexible tool for Java developers to build scalable, enterprise-grade applications, from customizing configurations to altering IoC behavior across environments.
The best way to learn to code? Code up some fun games from your childhood. Here's how to code the popular tic-tac-toe game in Java.
Want to know the difference between git fetch and pull commands? This quick pull vs. fetch comparison explains how the two commands affect your working directory differently.
The trickiest Java interview question ever asked? In five words or less, explain the red 'x' the Eclipse IDE displays at the end of the provided Java code snippet.
Want to learn how multiversion concurrency control works? With a very simple MVCC example, we'll explain how to eliminate locks in databases including PostgreSQL and etcd.
Need to learn Jenkins CI fast? This Jenkins tutorial will quickly get you up to speed on how to use the popular CI/CD tool.
Want to push a new project into an existing GitLab repository? Follow these steps, but beware one way that will work but might cause trouble on a shared repo.
Stop using the terms square brackets, round brackets and squiggly brackets. Use the terms braces, brackets and parenthesis instead. Here's the difference between them.
Developers need to name their git stashes and add a message if they want to easily identify them in the future. Here's how to pop and apply a specific Git stash by name.
Show your shelved change history with git stash list and show.
Here's a quick example that shows you how to rename a Git branch locally and remotely. The branch renaming commands and techniques work with GitHub, GitLab, Bitbucket and any cloud-based Git vendor.
Here are the three most common fixes to GitHub 'Permission denied (publickey)' SSH error.
The correct way to convert a String to long in Java is with the parseLong method of the wrapper class. The constructor conversion approach is deprecated! Use parseLong instead.
Want to unstage a file in Git? Use the restore command. Other options to have Git remove a file from the staging index have unintended consequences you'd really rather avoid.
Why is the const keyword in Java not implemented? It's part of the language, but it can't be used to make a variable constant. Here's why const is included in Java, and why Java's const keyword is ...
Here's a very quick example of how to setup GitHub SSH keys and use them to perform Git clone, push and pull commands over a securely configured SSH connection.
To connect Git operations securely to your GitLab repository, you will need to configure GitLab SSH keys both locally and remotely. Here's a quick example of how to do it.
This example shows you how you can find and use the GitHub URL. Clone, fetch, push and pull to your remote repo easily with the GitHub URL at your disposal.
Ubuntu, Linux and Windows Git configuration files are an unwieldy mess. Or at least is seems that way when you realize it's contained in three uniquely named files and spread across three or four ...
A common problem in computing is to find out if a list contains duplicate entries. It's also a good subject to explore various ways to solve a problem. In this case, we will see the performance ...
The incubating Java Vector API gives software developers access to fast and efficient SIMD operations. Here's an example of how to solve the prefix sum problem with SIMDs and Vectors.
Avoid Git's fatal 'unable to auto-detect email' error and set your username and email address in Git config. This global git config example shows you how.
Need to install the Eclipse IDE on Windows? This quick video tutorial shows you how to install Eclipse and build your very first Java application in the popular, open-source IDE.
Need to dedupe a Java list? Here are 5 simple ways to remove duplicates from a List in Java.
The prefix sum problem is often used to test a developer's mettle. Here's a simple solution to the prefix sum problem in Java that uses loops, arrays and basic Java syntax.
Deduping is a common part of data cleansing. Here are four simple ways to remove duplicates from lists in Java.
There's a new programming language named Mojo in the works that is set to revolutionize AI and ML while fixing all that's wrong with Python. Here is how to code your first Mojo program.
For enterprise systems that need a flexible, asynchronous and message driven architecture, the Actor Model is often the right fit.
The easiest way to undo a git commit is with the git commit --amend command. This quick tutorial will show you how to quickly amend any Git commit.
The Git index, along with the need to use the "git add" command before performing a commit, is often a source of confusion for beginners. These "git add" examples will make things clear.
To get started with Git, you need a git repository. This quick tutorial will show you how to use "git init" to quickly create a git repo on your local machine.
When you compare Java vs. Python application development in terms of simplicity, conciseness, security and performance, Java comes out on top in every category.
Python can't thread across cores, and the reason why dates back 30 years to a fatally flawed assumption that computers of the future would have only one CPU.
Need to install Git on Windows? This quick Git install tutorial shows you how to quickly download, install and configure Git on Windows 10.
There comes a time where you must clean up Git branches, commits and the repository as a whole. Here we show you how to compact your Git repo and keep things clean.
Need to git clone a specific commit? There's no single command to do it, but clever use of branch and reset commands makes it possible to clone a single, specific git commit.
Need to git clone a single, specific branch? In this quick example, we show you how to git clone a specific branch to help save your builds time and space.
Developers can use the git clean command to delete untracked files in a working tree and remove what they don't need from a local repository. Here is how to issue the command and some helpful tips ...
Don't overlook a file or two when you attempt to remove and discard local, uncommitted changes in Git. Learn how to locally revert and remove uncommitted changes to files in Git properly.
Did you create a new Git branch? Need to push that local Git branch to a remote repo like GitHub, BitBucket or GitLab? This example shows you how to git push new local branches remotely.
Who needs a GitKraken crash course? For those who want a top-tier Git GUI tool that can help accelerate software development, this GitKraken tutorial for beginners is for you.
Curious about the Kotlin language? It's an excellent choice as either a companion or alternative to Java, and offers many capabilities Java programmers wish they had all along.
If you start development before you set up version control, you may need to push your development project to an existing GitHub repo. This Git and GitHub example shows you the fastest way to ...
Need to learn Sourcetree and Bitbucket? This complete Bitbucket and Sourcetree tutorial will help you quickly master these industry leading Git tools.
With the Jenkins installer it's incredibly easy to download and run the popular CI/CD tool. Follow these simple steps and you'll have the continuous integration server running on Windows in no time.
Need to learn how to use GitHub Desktop? This quick and thorough GitHub Desktop tutorial for beginners will teach you all the fine points of source code management both locally and in the cloud.
Both hard and soft git reset commands manipulate the commit history. Where soft vs. hard git reset commands differ is by how they impact the index and the local filesystem.
There's no single Git command that will delete a remote and local Git branch. But these two commands will get the job done. Need to delete remote and local Git branches? Here's how to do it.
While there are almost 40 registered HTTP request methods, most RESTful web developers only use five of them. Here are the most important HTTP methods and examples of how to use them.
What's the difference between PUT and POST methods? Here we compare POST versus PUT in terms of idempotence, URL usage, payloads and their use in RESTful APIs.
Have you been snared by Docker's "unauthorized: incorrect username or password" error when you login at the command line? Here's how to generate an access token in Docker Hub and fix it.
The comparison of Scrum versus a sprint is less about differences and more about how Agile frameworks such as Scrum, SAFe and XP use short development cycles to limit risk and keep stakeholders ...
Want to quickly create an AWS Lambda function in Python? This quick Python and AWS tutorial shows how to develop, test and deploy your first Python Lambda function in just a few minutes.
Java 21 includes numerous highly anticipated preview features including String templates, scoped variables and unnamed classes. Here's how to enable Java 21 preview features on your JDK.
In a ham and eggs breakfast, the chicken is involved, but the pig's 100% committed. That's the problematic basis of Scrum's chicken and pigs parable.
There's something hubristic and self-effacing about issuing "sudo" commands. That's why I always say "please" instead -- and you should, too. Here's how to set "please" as an alias for "sudo" on ...
If you haven't created a Java-based AWS Lambda function before, you'll be amazed at how easy it is. This quick AWS, Lambda and Java tutorial will quickly get you started in the world of serverless ...
While the use of var in Java brings inferred typing to the platform, it does not negate Java's dedication to strong typing. Here's how the Java var keyword works, along with simple examples of how ...
How writing code on a Commodore 64 for fun led to a real-world optimization.
There are no shortcuts to passing the Scrum master certification exam, but there is one trick that will help confident test takers get closer to a perfect 100% score.
Say goodbye to the public static void main method in Java 21 with the unnamed classes and instance main methods preview features.
Effective Hibernate and JPA column mapping requires the use of the @Column annotation to set important properties such as nullability, uniqueness, precision and scale.
New developers are often confused by the line of System.out.println() code they write when first introduced to Java. Here's a quick explanation of what System.out.println() means.
Need to use raw SQL with JPA and JPA? The Jakarta Persistence API's NativeQuery interface makes it possible to use Hibernate, SQL and JPA together in the same app.
What's the difference between print and println methods in Java? Our examples show that it comes down to the fact that println adds a newline character to output, while Java's print method does not.
Is the daily Scrum recommended for colocated teams? Yes, yes it is. Any suggestion that the daily Scrum is not recommended for colocated teams is false.
Struggling to get your first JPA 3 application off the ground? Here's a quick Hibernate and JPA 3 CRUD example to help you get going with the latest version of the Java Persistence API.
The length of an Agile sprint is a topic development teams must figure out on their own, but from the Scrum perspective, Agile sprints should never go longer than a month.
No persistence.xml file found fix The hardest part about learning Hibernate and JPA 3 is how to get your first project up and running. Some will encounter the dreaded no persistence.xml file found ...